Are you looking to optimize your eCommerce performance? A/B and multivariate testing offers is a powerful tool in improving effectiveness.
Saying goodbye to guesswork, this approach gives you valuable insights to make data-driven decisions about how best to get the most out of each offer without wasting time or money.
Why run A/B and multivariate tests?
In the world of eCommerce, every marketing decision counts. That's where A/B and multivariate testing are helpful - they allow you to experiment with different strategies to find the best possible outcome.
- A/B testing involves comparing two versions of a web page or email to see which performs better.
- Multivariate testing allows businesses to test multiple variables at once.
Both types of testing involve optimization through trial and error and can provide invaluable insight into customer behavior.
Additionally, A/B and multivariate testing give you valuable insights into customer behavior and preferences, allowing you to tailor your approach better to meet your audience's needs.
Testing and analyzing the results can have very profitable outcomes. Recently, RevLifter helped US Polo Assn test the difference between discounts at '$ off' vs. '% off'. While there was no uplift for one over the other, the '$ off' resulted in more profitable sales. Due to this, the brand started running more '$ off' promotions.
A/B and multivariate testing in the RevLifter platform
The RevLifter platform is the perfect place to conduct different tests to see what works. These are some of the areas we'd focus on:
Offer types
For example:
- 5% vs 10% vs 15% off
- 10% off vs £15 off
- Upsell offers vs cross-sell offers
- With a progress bar vs no progress bar
- With product recommendations vs with no recommendations
- 'Frequently bought with' recommendation vs 'people also viewed' recommendation
Offer copy
Test different variations of the offer copy, such as the selection or placement of keywords.
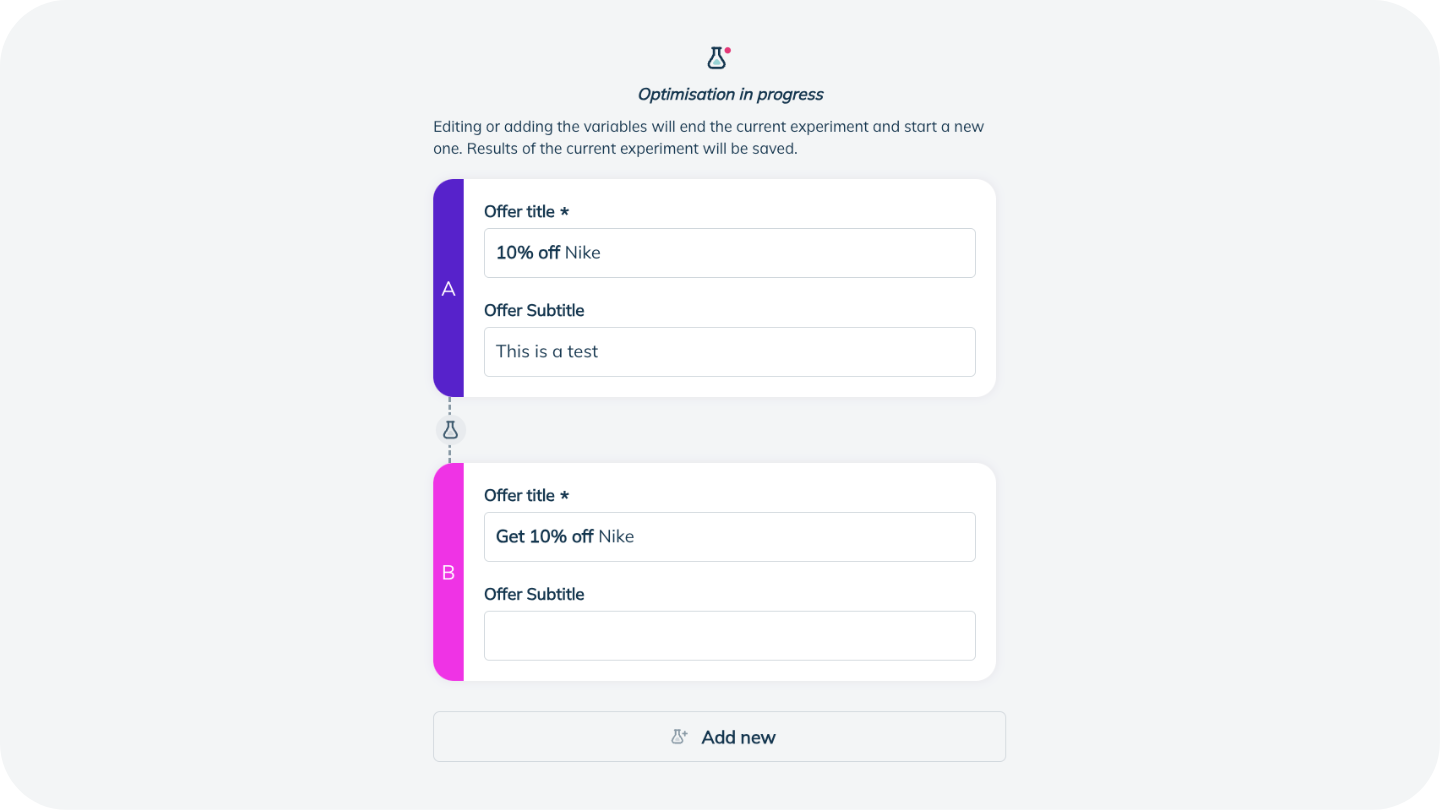
Offer images
Test different offer images to see which drives more engagement and sales, for example, a product vs a lifestyle photo.
Equal splits or auto-optimization?
Your next step is to choose how your experiments should work. You can choose to run experiments on an even split or have them auto-optimize based on the selected objective.
Running an equal split
Selecting a split test will show the offer variables to equal proportions of traffic. For example, if four variables exist, each variable will be delivered to 25% of the eligible audience.
Auto-optimization using machine learning
We use a machine learning algorithm to choose the best variation at that moment based on the available data. This automatically weights the winning objective to be shown to most traffic based on your goals, including:
- Conversion rate (CVR)
- Average Order Value (AOV)
- Average Revenue Per User (ARPU)
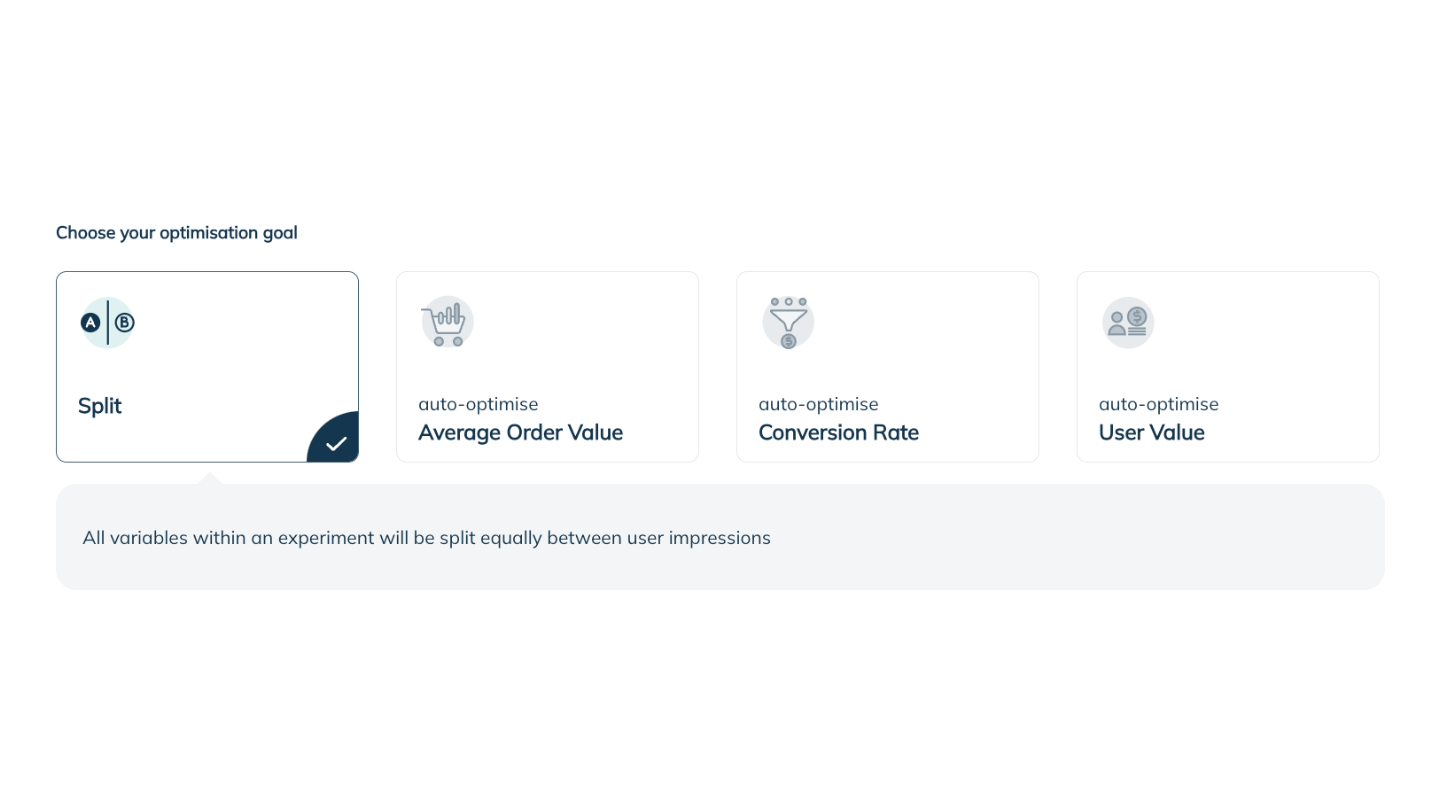
If one variable outperforms all the others, our experimentation engine automatically shows the winning variable to a more significant percentage of your traffic.
It continually weights them and does so in a way to minimize "regret" for showing the wrong offer or variant. If two variations are higher than the others, it will lean into them.
Similarly, if the variations are very close but slightly different, it will show them roughly evenly but lean towards the one that performs the best.
Tracking testing results
With RevLifter, it's incredibly easy to track your performance and see which variables are winning out. In the experiment report below, you can see the following:
- An overview showing which offer or campaign the experiment belongs to and what the objective is.
- A performance table that shows results by each variant.
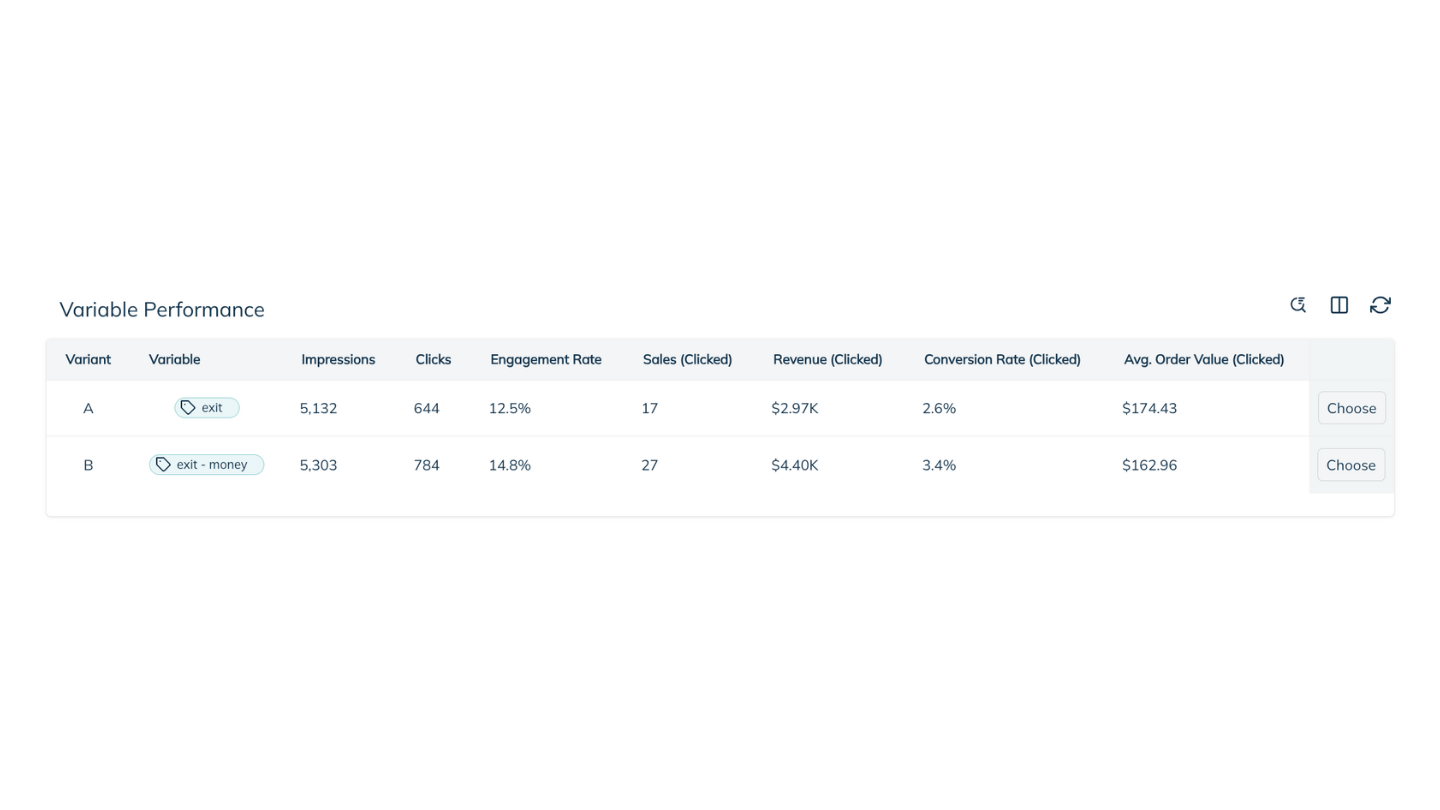
Try A/B and multivariate testing yourself!
A/B and multivariate testing provide a strong foundation for eCommerce optimization, allowing you to test, learn, and adapt your strategies based on data-driven insights.
They facilitate an understanding of customer behavior and preferences, which in turn guides the tailoring of offers and the enhancement of customer engagement.
The RevLifter platform uses both types of testing, coupled with machine learning for auto-optimization, to continually improve offer performance. By employing these strategies, you can maximize your conversion rates, average order values, and average revenue per user.
Get a demo to learn more about testing in the RevLifter platform.